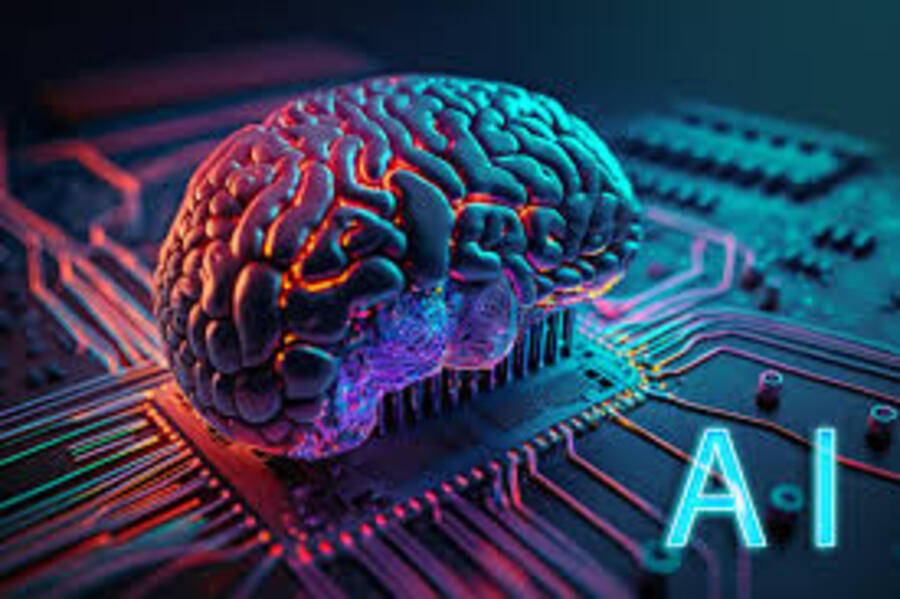
Artificial intelligence is advancing at a breakneck pace, and 2024 is seeing some significant trends. Here’s a look at the most prominent AI trends shaping various industries and how they’re impacting our day-to-day lives:
1. Generative AI and Multimodal Systems
- Generative AI has expanded beyond text and image generation to handle multimodal inputs (text, images, audio, video), enabling richer, more intuitive interactions.
- Applications: From digital content creation and marketing to personalized media, healthcare, and complex simulations, generative AI is being widely adopted. Multimodal AIs that can simultaneously analyze video, text, and audio in real time are becoming useful for applications like virtual training environments, customer service, and immersive AR/VR experiences.
2. Responsible AI and Ethical AI Frameworks
- As generative AI and deep learning applications proliferate, Responsible AI is becoming a cornerstone for enterprises and governments alike. Ethical AI frameworks aim to ensure transparency, fairness, and compliance with regulations, such as data privacy and cybersecurity laws.
- Applications: Organizations are now employing transparency in model explanations, data sources, and the ethics of AI decisions. Industries like finance, healthcare, and law, where decision-making has far-reaching consequences, are leading this trend.
3. AI for Drug Discovery and Healthcare Innovation
- In healthcare, AI has become a powerful tool for drug discovery, diagnostics, and personalized treatment plans. With recent breakthroughs, AI is accelerating drug discovery timelines, identifying new therapeutic targets, and predicting patient responses to treatments.
- Applications: Generative models for drug molecule creation, AI-enhanced diagnostic tools, and customized treatment algorithms are transforming healthcare. Hospitals and clinics are leveraging AI to optimize workflows, personalize patient care, and predict patient outcomes with more accuracy.
4. AI-Driven Automation in Software Development (AutoML and Code Generation)
- AI is now making a mark in software development with tools for automated coding, debugging, and optimization. AutoML (Automated Machine Learning) solutions help automate repetitive tasks in model training and testing, while code generation models speed up the development cycle.
- Applications: Tech teams are using these tools for faster prototyping, debugging, and even full-cycle development. Startups and large tech companies are embracing these to reduce the cost and complexity of developing high-quality software.
5. AI in Cybersecurity
- The rise of AI-enhanced cyberattacks has driven companies to adopt AI for advanced threat detection and response. AI models can recognize patterns in real-time, detect anomalies, and anticipate potential breaches by analyzing network traffic and user behavior.
- Applications: Automated systems are now integral for risk assessment, vulnerability management, and incident response. Financial institutions, governments, and large corporations are prioritizing AI-driven security measures to protect sensitive data and infrastructure.
6. Federated and Privacy-Preserving AI
- Privacy concerns have led to the development of federated learning and other privacy-preserving AI approaches that allow models to train on decentralized data without it leaving the source device or network.
- Applications: Mobile apps, healthcare platforms, and IoT devices are leveraging federated learning to enhance user privacy while still benefiting from AI insights. Companies in regulated sectors are adopting these methods to comply with data privacy laws.
7. Edge AI and IoT Integration
- With the proliferation of IoT devices, Edge AI is emerging as a necessity for processing data close to the source rather than relying solely on cloud computation.
- Applications: Smart cities, autonomous vehicles, and industrial automation are using Edge AI to make real-time, on-device decisions. Edge AI lowers latency and improves performance, enabling faster, localized decision-making for applications where speed is critical.
8. Specialized AI Chips and Hardware Acceleration
- The demand for faster and more efficient AI computations has led to specialized AI chips, such as GPUs, TPUs, and NPUs, designed to optimize processing in both training and inference tasks.
- Applications: From autonomous vehicles to drones, data centers, and high-performance computing, these chips are enabling more efficient AI workloads. As these chips become more energy-efficient, they’re also aiding in the development of environmentally sustainable AI solutions.
9. AI-Enhanced AR and VR Experiences
- AI is enriching augmented reality (AR) and virtual reality (VR) experiences, particularly in entertainment, gaming, and training applications. With AI, AR/VR can deliver more immersive and interactive experiences by adapting to user behavior.
- Applications: AI-driven avatars, interactive learning environments, and digital twins are gaining traction. Retail, real estate, and remote training environments are particularly benefitting from these technologies.
10. Natural Language Processing and Conversational AI Advancements
- NLP advancements have enabled AI to understand and generate more contextually relevant responses in conversational interfaces. Enhanced understanding of context, intent, and sentiment makes AI interactions feel more natural.
- Applications: Improved virtual assistants and chatbots are becoming integral to customer service, sales, and employee assistance platforms. These conversational AIs are now capable of handling complex dialogues, making them valuable in healthcare, education, and customer support.
11. Quantum AI and Hybrid Models
- The fusion of quantum computing and AI has become a hot area of research, aiming to solve complex optimization and data processing problems that are impractical for classical computers.
- Applications: While still in early stages, quantum-enhanced machine learning holds potential for industries needing massive processing power, like finance, logistics, and drug discovery. Hybrid AI models that combine quantum and classical computing are being researched for breakthroughs in these fields.
12. Sustainable AI and Green Computing Initiatives
- AI research is increasingly focusing on energy-efficient algorithms and sustainable practices to reduce the environmental footprint of large AI models, especially those that require extensive computing resources.
- Applications: Companies are adopting practices such as carbon offsetting, using green data centers, and implementing energy-efficient models. Environmental monitoring systems, as well as industries with high energy consumption, are at the forefront of this trend.
13. Digital Twins in Manufacturing and Beyond
- AI-powered digital twins are being used to create virtual replicas of physical assets for monitoring, simulation, and optimization. They are particularly useful in industries like manufacturing, construction, and aerospace.
- Applications: Manufacturers and energy companies use digital twins for predictive maintenance and process optimization, while urban planners employ them for sustainable city planning. Digital twins help reduce costs, improve productivity, and ensure safety by providing real-time insights into complex systems.
2024’s AI landscape is marked by a balance between innovation and responsibility, with applications spanning from everyday devices to the most complex scientific challenges. These trends indicate a strong push toward integrating AI in ways that are ethical, efficient, and sustainable across industries.