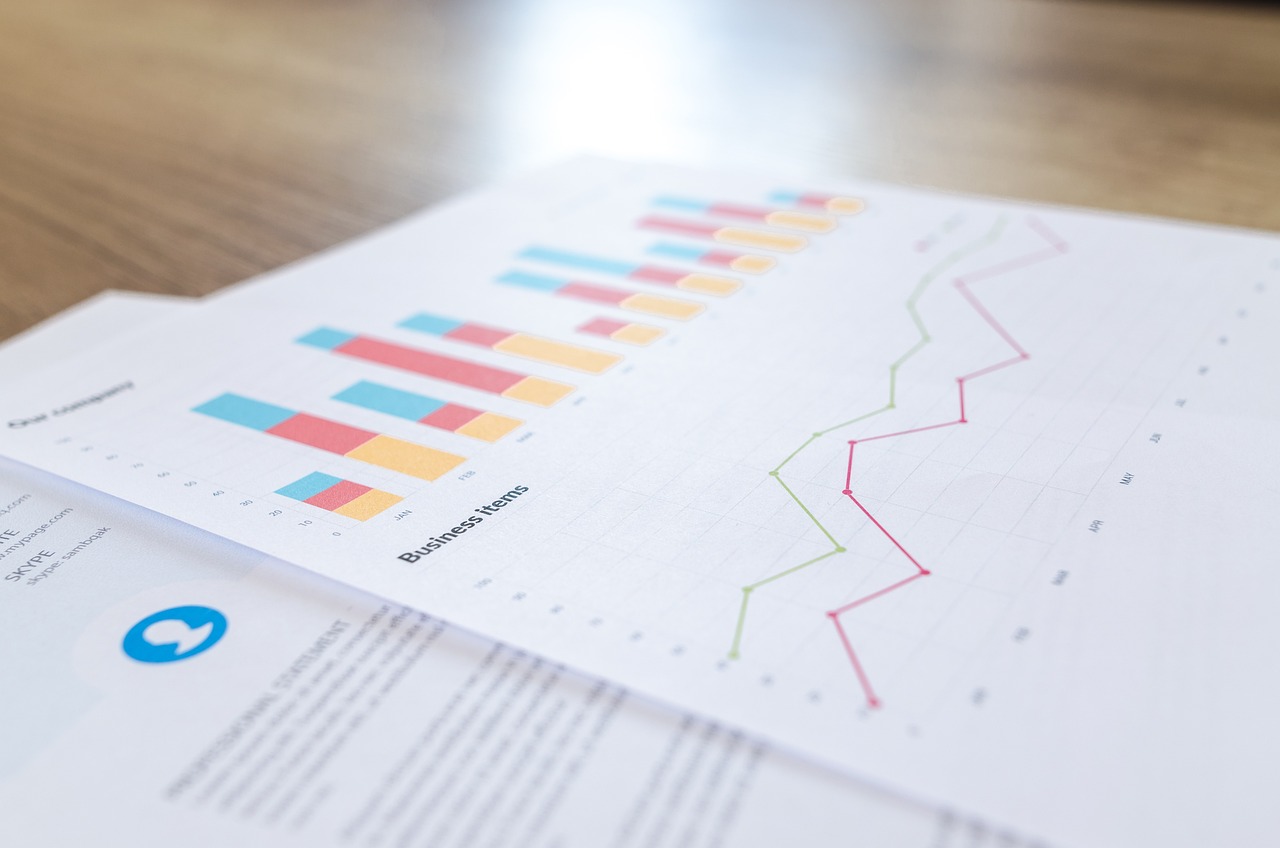
Introduction
In an increasingly competitive and volatile market, companies are always searching for ways to predict their financial future, navigate risks, and maximize growth opportunities. One powerful tool that allows companies to do this is forecasting business health through historical financial data. By analyzing historical financial trends, businesses can build predictive models that provide insights into future performance, potential risks, and resilience in the face of market fluctuations.
Today, with advancements in artificial intelligence (AI), especially for loan companies, predictive analysis is becoming even more accurate and comprehensive. AI-powered analytics can process large volumes of historical financial data, enhancing the forecasting capabilities of financial institutions and giving loan providers crucial insights into borrower behavior and financial trends. This article will delve into how historical financial data can be leveraged to predict future business health, with a focus on the role of AI in facilitating this process.
Understanding Historical Financial Trends
Historical financial data consists of information on a company’s previous financial performance, typically documented in analyzing the financial statements such as income statements, balance sheets, and cash flow statements. These documents reveal patterns and trends across different periods, providing valuable insights into a company’s profitability, liquidity, solvency, and overall financial health. By assessing these patterns, companies can better understand their strengths, weaknesses, and growth potential.
Common financial metrics examined in historical trend analysis include:
- Revenue growth rates
- Profit margins
- Debt-to-equity ratios
- Asset turnover rates
- Liquidity ratios (such as current and quick ratios)
- Cash flow trends
For instance, if a company has a steady revenue growth rate, stable profit margins, and a manageable debt-to-equity ratio over time, it may be considered financially healthy and capable of sustaining growth. Conversely, declining revenue, shrinking profit margins, and rising debt levels might indicate financial distress and potential risks.
Building Predictive Models from Historical Data
To leverage historical data effectively, companies can use various statistical techniques and predictive models, which can range from simple linear regression models to complex machine learning algorithms. Here’s a breakdown of how these models can use historical data to forecast future business health:
- Trend Analysis and Projection: This method involves examining trends in historical data to predict future outcomes. For example, a company with a consistent annual revenue growth rate of 5% may project similar growth if market conditions remain stable. This technique, although simplistic, is helpful in setting baseline expectations.
- Regression Analysis: Regression models can identify relationships between various financial factors and predict future outcomes based on these relationships. For example, an analyst might use regression to explore the relationship between marketing expenditure and revenue growth, which can be valuable for companies looking to optimize spending.
- Time Series Analysis: Time series forecasting techniques, such as moving averages, autoregressive integrated moving average (ARIMA), and exponential smoothing, are commonly used to analyze historical data. Time series models are particularly effective for analyzing seasonal data, helping companies plan around cyclical fluctuations.
- Machine Learning Models: Modern machine learning techniques, such as neural networks and decision trees, can process large datasets and uncover complex patterns that are often missed by traditional models. AI can enhance these models by processing vast historical data with minimal human intervention, yielding high accuracy and precision in forecasting.
AI-Powered Predictive Analysis for Loan Companies
The use of AI for loan companies has revolutionized financial forecasting, especially for lenders who rely on precise insights to evaluate borrower creditworthiness and portfolio risks. AI-driven analytics allow loan providers to process extensive historical data, including borrower payment histories, economic trends, and market fluctuations, to predict defaults, assess credit risk, and optimize loan terms.
Key applications of AI in predictive analysis for loan companies include:
- Credit Scoring: AI models use historical data on borrower behaviors to build detailed credit scores. These models can identify patterns in repayment behavior and assess risk levels more accurately than traditional scoring methods.
- Default Prediction: Machine learning algorithms can analyze historical financial and transactional data to detect potential default risks. This allows loan companies to take proactive measures, such as adjusting loan terms or implementing enhanced monitoring, to mitigate potential losses.
- Loan Pricing Optimization: AI can help set optimal interest rates based on historical and predicted trends. By factoring in borrower profiles, economic conditions, and market risks, AI models can ensure that loan pricing remains competitive and profitable.
- Portfolio Risk Assessment: For loan companies managing multiple loans, AI can analyze historical performance data to forecast portfolio risks. This helps institutions manage their overall risk exposure and allocate resources more effectively.
Leveraging AI for Enhanced Predictive Accuracy
AI-powered tools can rapidly process complex financial data, identify trends, and generate forecasts that would be otherwise difficult or impossible to achieve manually. Some ways AI enhances forecasting accuracy include:
- Data Processing and Integration: AI tools can handle vast amounts of data from various sources, such as market data, economic indicators, and borrower records, allowing for comprehensive trend analysis. This data integration helps loan companies and other financial institutions gain a more holistic view of financial trends and borrower profiles.
- Advanced Pattern Recognition: Machine learning algorithms excel at identifying patterns within large datasets. These algorithms can pinpoint correlations and causal relationships that traditional analysis might miss. For example, AI might recognize that certain borrower behaviors correlate strongly with repayment defaults.
- Real-Time Updates: AI models can continuously update forecasts as new data becomes available. This real-time analysis is particularly valuable for loan companies that need to respond swiftly to changing market conditions or borrower behaviors.
- Improved Decision-Making: By providing accurate, data-driven insights, AI-powered predictive models can enhance decision-making across departments. Financial institutions can use these insights for risk assessment, investment strategy, and customer relationship management.
Case Study: Predicting Financial Health in Practice
Consider a hypothetical case of a loan company that wants to forecast the likelihood of defaults within its loan portfolio over the next year. The company begins by gathering historical financial data on its borrowers, including payment histories, debt levels, and income patterns.
Using this data, the loan company employs a machine learning model designed to detect patterns indicative of default. The model identifies variables, such as frequent late payments or rising debt-to-income ratios, as risk factors. With AI, the company also incorporates external data, such as changes in unemployment rates and inflation, which impact borrowers’ repayment capabilities.
The predictive model then generates a score for each borrower, indicating their likelihood of default. This scoring helps the company adjust loan terms for high-risk borrowers, allocate funds for debt recovery, and refine its overall lending strategy. By relying on historical data and AI-powered predictive analysis, the loan company can mitigate risk and enhance portfolio performance.
Benefits of Historical Financial Trend Analysis for Business Health Forecasting
Using historical financial trends for predictive analysis provides several key advantages:
- Proactive Risk Management: Identifying potential risks early allows companies to take proactive steps, such as restructuring debt or adjusting operational strategies, to mitigate negative impacts.
- Informed Strategic Planning: Forecasting based on historical data helps businesses develop more accurate financial projections, which in turn supports strategic planning and budgeting.
- Enhanced Investor Confidence: Transparent and data-driven forecasting boosts investor confidence, as stakeholders can see a company’s resilience and commitment to long-term success.
- Efficient Resource Allocation: Predictive analysis helps companies allocate resources where they are most likely to yield a positive return, enhancing overall operational efficiency.
Challenges and Limitations
Despite the benefits, forecasting based on historical data does come with certain challenges:
- Data Quality: Inaccurate or incomplete historical data can lead to misleading forecasts, so it’s essential to ensure data accuracy and consistency.
- Market Volatility: Sudden shifts in the economy or industry can render past trends less relevant. For instance, unprecedented events like the COVID-19 pandemic disrupted typical financial patterns.
- Bias in AI Models: AI models are only as good as the data they are trained on. If historical data includes biases, the predictive model may reinforce these biases, leading to skewed predictions.
The Future of Financial Forecasting with AI
The integration of AI in financial forecasting continues to evolve, particularly in the loan industry. As technology advances, we can expect AI tools to become more accurate, adaptive, and user-friendly, enabling financial institutions to refine their predictive analysis even further.
Future developments may include more sophisticated AI models capable of adjusting to sudden market changes, as well as improved tools for interpreting and visualizing complex financial forecasts. Additionally, the growing importance of ESG (Environmental, Social, and Governance) considerations may see AI tools incorporating these factors into financial predictions, aligning financial forecasting with sustainable business practices.
Conclusion
Leveraging historical financial trends for predictive analysis provides businesses with a roadmap for future performance, helping them make strategic decisions and manage risks effectively. With the support of AI, particularly in loan companies, the accuracy and scope of these predictions have greatly improved. By adopting AI-driven predictive models, businesses can anticipate challenges, seize opportunities, and build a resilient financial foundation for the future.